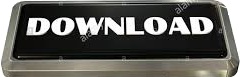
Causes of resource scarcity vary but can include poor access to technology for developing these resources, a relatively small population of speakers, or a lack of urgency for collecting such resources in bilingual populations where the second language is high-resource.

Languages are classified as low-resource when they lack the quantity of data necessary for training statistical and machine learning tools and models. Through these interventions, we not only created a refined and evaluated corpus of 26,240 Hindi-Gondi translations that was used for building the translation model but also engaged nearly 850 community members who can help take Gondi onto the internet. We also present preliminary evaluations of utilizing the developed machine translation model to provide assistance to volunteers who are involved in collecting more data for the target language. During this process, we help expand information access in Gondi across 2 different dimensions (a) The creation of linguistic resources that can be used by the community, such as a dictionary, children's stories, Gondi translations from multiple sources and an Interactive Voice Response (IVR) based mass awareness platform (b) Enabling its use in the digital domain by developing a Hindi-Gondi machine translation model, which is compressed by nearly 4 times to enable it's edge deployment on low-resource edge devices and in areas of little to no internet connectivity. In this paper, we report the deployment of technology-driven data collection methods for creating a corpus of more than 60,000 translations from Hindi to Gondi, a low-resource vulnerable language spoken by around 2.3 million tribal people in south and central India. This work is licensed under a BSD 3-Clause License.The primary obstacle to developing technologies for low-resource languages is the lack of representative, usable data. For major changes, please open an issue first to discuss what you would like to change. If you have a project that you would like included in this list, please open an issue, submit a pull request or contact me at Contributing The service is an interface to Deezer's Spleeter stem separation library that uses Tensorflow with pretrained models written in Python. Spleeter Online extracts the vocal stem from any mp3 or wav file. Projects Spleeter Online - spleeter.online This is made possible by an included Globa Media Control Service RXJS behavior subject that the components listen to for notification to stop when another player is going to play. When playing any waveform rendererd with this service, all other players instantiated by this service will stop.
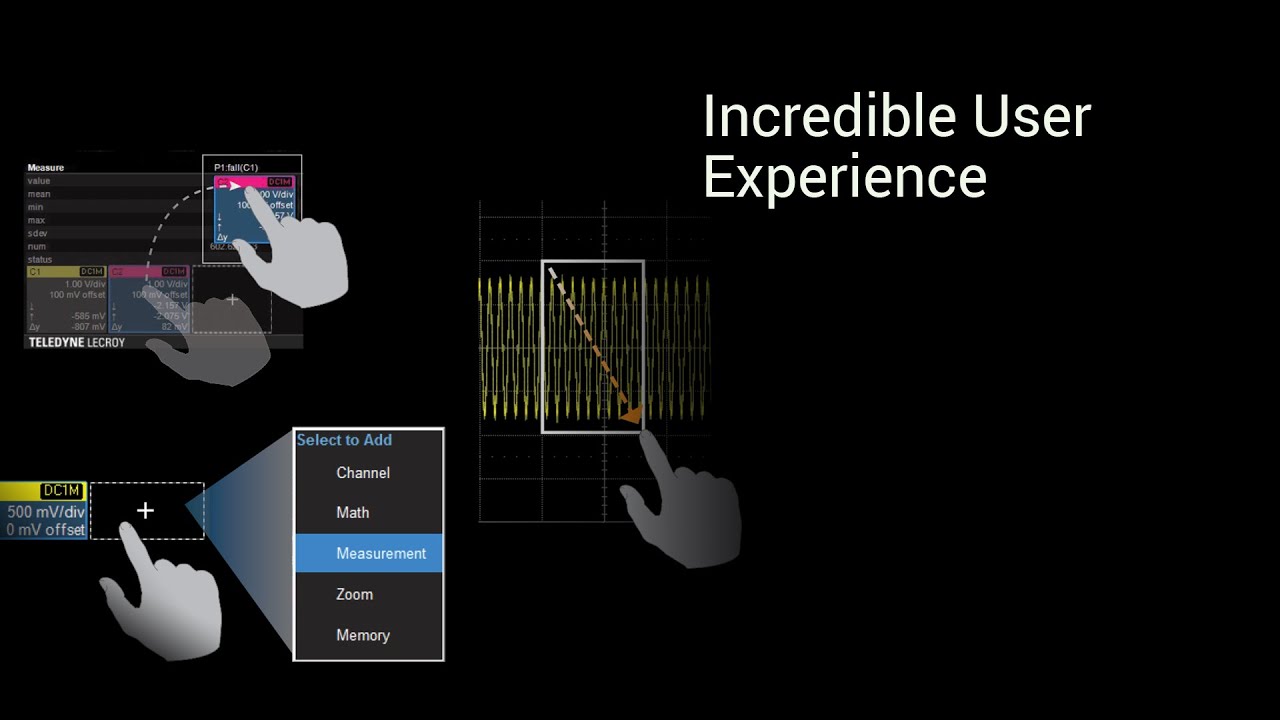
import ) Īll rendered waveforms are click-to-play.
WAVESURFER ANGULAR2 INSTALL
npm install angular-wavesurfer-service -saveĪdd AngularWavesurferServiceModule to your NgModule. Use your favorite package manager / npm to install. Player with Controls - Play, pause and stopĭynamic Control Classes - Add classes to indicate the playback stateĬurrent Time Display - Display the current progress of playback
WAVESURFER ANGULAR2 CODE
Examples WaveServiceīase Template - Minimum required code to get up and running
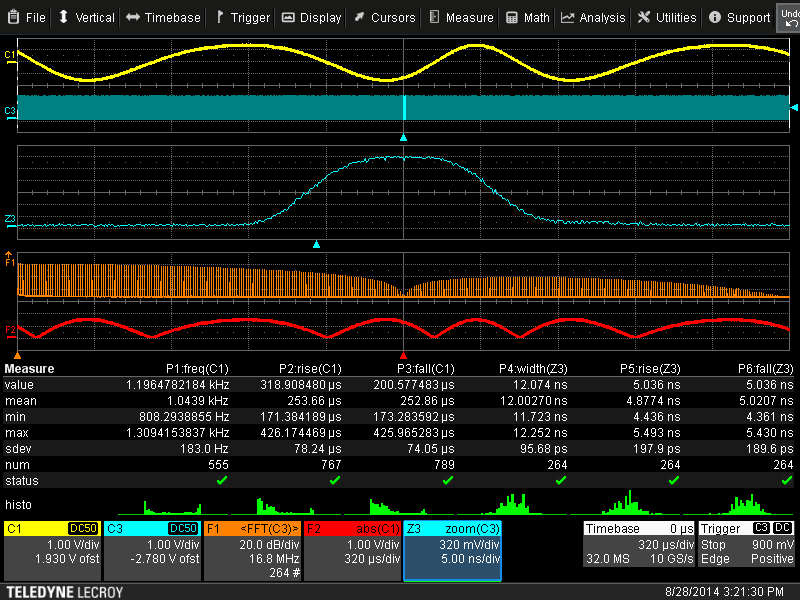
With wavesurfer.js you can create anything from an HTML5 audio player to a sophisticated DJ application. Wavesurfer.js is a customizable audio waveform visualization, built on top of Web Audio API and HTML5 Canvas. This library provides wavesurfer.js as an Angular service and component.
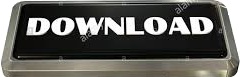